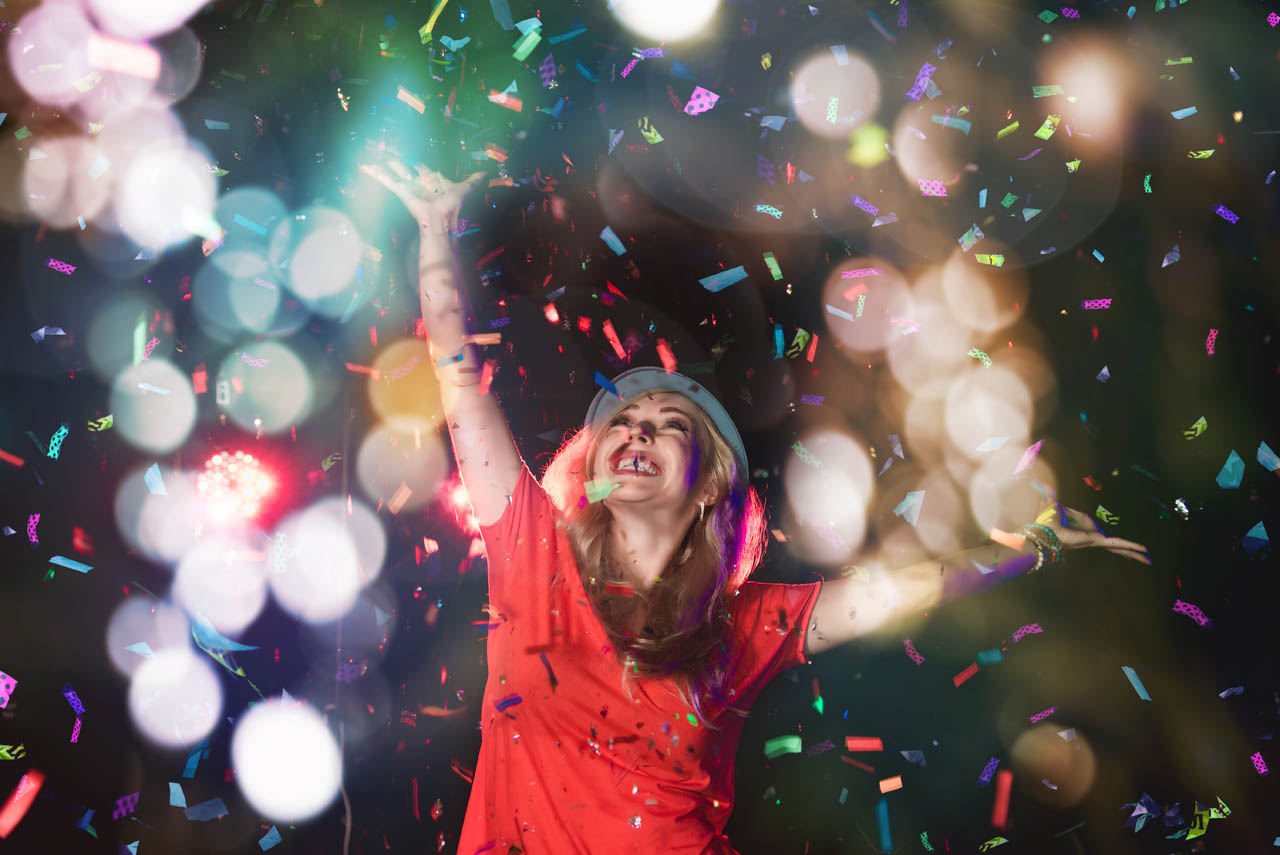
Decoding High-Stakes Innovation in Modern Technology
In today’s rapidly evolving tech landscape, innovative models such as Amazon Spin & Win serve as a nexus for integrating strategic financial mechanisms with cutting-edge technologies like AI and Big Data. This article explores the pivotal elements of payout structures, confidence level analytics, maximum risk assessments, steady progression strategies, tiered bonuses, and safehedging—each vital to developing robust, data-driven decision frameworks (Brynjolfsson & McAfee, 2014).
Technological Underpinnings and Strategic Implications
Key to understanding these systems is the dynamic interplay between algorithmic risk analysis and modern simulation techniques. Companies are increasingly leveraging AI-enhanced models to forecast payout outcomes under various market conditions, ensuring maximum risk remains a controlled variable. The concept of steady progression underscores the necessity for incremental advancements in system robustness, while tiered bonus strategies incentivize nurturing AI confidence levels and risk-mitigated behaviors.
Operational Mechanisms: From Theory to Practice
The revered theory behind safehedging strategies finds its roots in both traditional financial hedging (Hull, 2015) and recent advancements in predictive analytics. By combining tiered rebates and risk shields into a coherent operational framework, stakeholders can achieve a symbiotic relationship between high reward payout potentials and rigorous risk management protocols. This model paves the way for maximizing confidence levels through transparent data flows and steady progression practices.
Frequently Asked Questions (FAQs)
Q1: How does the Amazon Spin & Win model integrate AI with risk management?
A1: It incorporates AI algorithms to assess payout probabilities and optimize risk control measures, ensuring rapid adaptation to market fluctuations.
Q2: What role does Big Data play in enhancing the confidence level?
A2: Big Data provides the volume and variety of information needed to validate model assumptions, enabling precise adjustments in tiered bonus structures and safehedging strategies.
Q3: Can these systems adapt to dramatic market changes?
A3: Yes, through dynamic calibration methods and steady progression protocols supported by real-time analytics, the framework remains resilient amidst unexpected market volatility.
Interactive Discussion
What are your thoughts on integrating advanced AI techniques into traditional risk management frameworks? Do you think steady progression in payout models could counterbalance high market risks effectively? We invite you to share your opinions and cast your vote on which component—confidence level analytics or tiered bonus strategies—plays a pivotal role in modern fintech innovations.
Comments
Alice
The blend of theoretical risk models with practical AI applications is fascinating. Looking forward to more insights on safehedging strategies.
小伟
文章内容详尽,不仅讲解了技术细节,还对现代科技的发展给予了高度评价,非常有启发性!
JohnDoe
This article is a great resource on merging traditional finance with AI. The breakdown on tiered bonus mechanisms was particularly enlightening.
明华
很高端的分析,对于如何利用大数据来提升风险控制能力有很好的阐释。