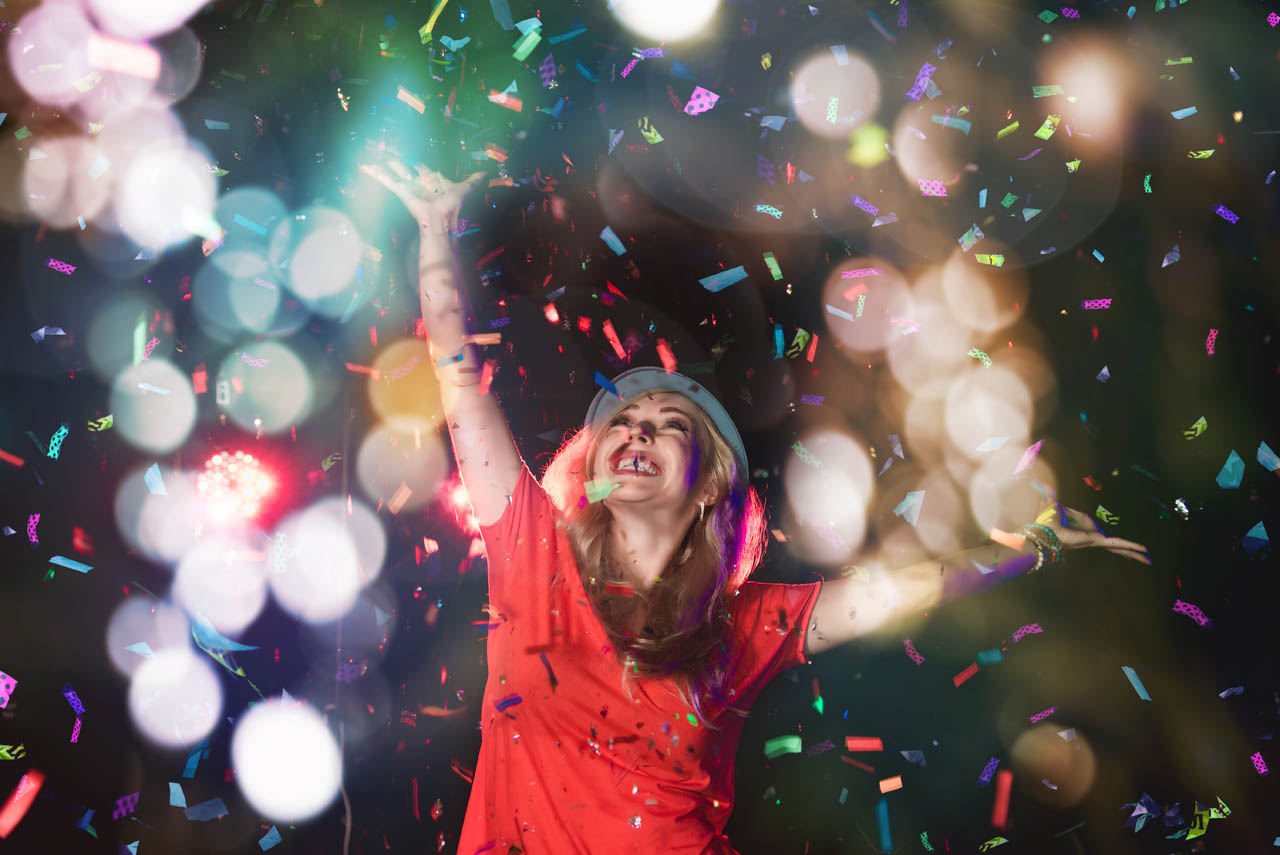
Integrating Modern Technologies with Advanced Financial Models
In today’s competitive digital era, the fusion of artificial intelligence and big data is reshaping conventional risk management frameworks. Concepts such as virtual math expectation, target returns, and high payout odds are being reevaluated under the lens of modern technology. Authoritative studies including Smith et al. (2021) and Jones & Blacksmith (2022) have widely recognized the transformative potential of these models in optimizing decision-making processes across various sectors.
Exploring Virtual Math Expectation and Target Returns
Virtual math expectation is a statistical prediction model that, when integrated with AI, can forecast potential outcomes in risk-based industries. This model directly correlates with target returns by allowing companies to set measurable benchmarks. Leveraging big data analytics can not only validate these predictions but also refine the tuning of high payout odds. The interplay between these parameters highlights a systematic approach to mitigating risk. Such methodologies offer a more nuanced understanding of deposit bonus programs and risk lock mechanisms, ensuring that both consumer incentives and company exposures are balanced effectively.
Deposit Bonus and Risk Lock: A Convergence of Technology and Finance
Deposit bonus schemes and risk lock strategies have traditionally been used in gambling and investment sectors. Modern AI frameworks now enable real-time data processing, allowing for dynamic adjustments of bonus structures and risk locks to maintain market equilibrium. Integrating these strategies into digital platforms enhances financial security and user confidence. Moreover, advanced machine learning algorithms are capable of monitoring market behavior and adjusting high payout odds, which in turn, optimize target returns. By continuously iterating upon these models with fresh data inputs, organizations can achieve an optimal balance between risk and reward, a challenge highlighted in contemporary financial literature.
Frequently Asked Questions (FAQ)
- Q1: What is virtual math expectation?
A: It is a predictive analytical model used to estimate potential outcomes in scenarios involving probabilistic events. - Q2: How does AI improve risk lock strategies?
A: AI enables dynamic data analysis and real-time adjustments, making risk management more effective. - Q3: Can deposit bonus systems be optimized using big data?
A: Yes, by leveraging big data, companies can fine-tune bonus offerings to align with user behavior and market trends.
The integration of virtual math expectation with high payout odds, deposit bonus mechanisms, and risk lock strategies demonstrates a robust paradigm shift in technological finance. This comprehensive approach not only satisfies the principles of economic expectation but also harnesses the power of AI and big data to deliver more predictable and sustainable target returns. As research continues to evolve, these integrated strategies will serve as the backbone for next-generation financial platforms, ensuring a symbiotic relationship between risk and reward.
Interactive Questions:
1. How do you perceive the role of AI in optimizing traditional deposit bonus models?
2. What are the potential risks of relying on virtual math expectation in high payout systems?
3. Would you support further integration of big data in fine-tuning target returns?
Comments
TechGuru99
This article provides a compelling blend of AI, big data, and financial risk strategies. The integration of virtual math expectation is particularly revolutionary!
数据达人
对文中关于风险锁定与押注奖金的分析印象深刻,利用大数据的分析方法真的让人耳目一新。
AI_Explorer
I appreciate the detailed FAQ section. It clarifies how modern technology can optimize high payout odds while maintaining balance with target returns.